Eduard Porta: The future of Artificial Intelligence in biomedicine is bright
Dr. Eduard Porta, leader of the Cancer Immunogenomics group at the Josep Carreras Leukaemia Research Institute, has participated in a community initiative to put into context the value of AlphaFold2 predictions, an algorithm created by Deep Mind, the Google's specialized artificial intelligence company, capable of determining the three-dimensional structure of all known human proteins. Their conclusions have recently been published in the specialized journal Nature Structural Biology.
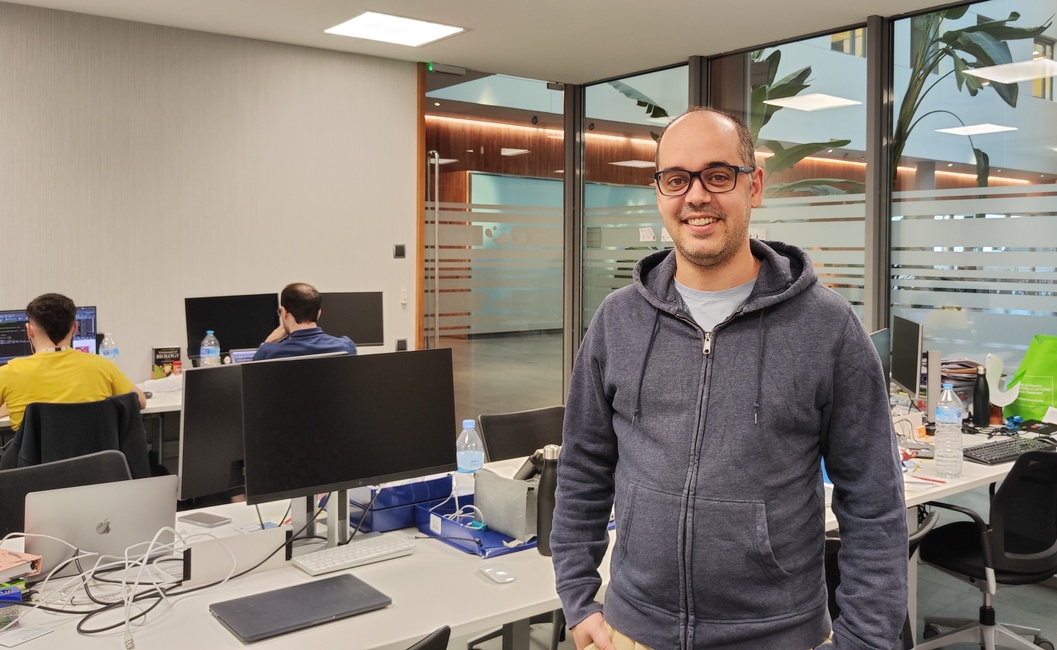
In this conversation, Dr. Porta explains the importance of having these 3D models and tells us that we are facing a new era, in which tools based on artificial intelligence will be the new standard in the laboratory.
Let's start from the beginning: what is a protein and why is it important to know their three-dimensional structure?
Proteins are molecules found inside cells that perform a large part of the functions that cells need to live. The shape they take in space is very important to perform these functions and they stop working if they don't fold correctly or take a different shape due to mutations, for instance. This can lead to very diverse diseases, such as cancer.
I see... so knowing the 3D structure of proteins can help find new therapies. How has it been possible to determine the 3D structure of all human proteins?
Well, with many years of work! For the last 70 years, scientists have been using expensive and time-consuming technologies such as X-ray crystallography or nuclear magnetic resonance. Until 2019, the structure of around 5,000 proteins had been achieved, out of the nearly 20,000 that exist in the human proteome.
There were 15,000 left still, and there are proteins that cannot be determined with these procedures so, for a long time, a way to predict their structure by computational means has been sought. In the mid-80s of the 20th century, the first algorithms working by similarity were generated. The idea behind was that proteins with similar sequence would have a similar 3D structure. This increased our knowledge to about 8,000 structures, but many were still missing.
And that's when Google comes into play, right?
Yes, in 2019 Google decided to participate in the international CASP contest, which is held every two years and brings together the entire community dedicated to the computational prediction of protein structures. His approach relies on neural networks and artificial intelligence and, to everyone's surprise – they are actually a computer services company and had never done anything in biology before -, he wins. And he wins by far, a long way from the second classified!
And the legend of AlphaFold begins, right?
Well, not exactly because, although they present many new structures, they do so without revealing their secret weapon and therefore the research community sees that there is a solution, but they do not have the tool and it is a bit disappointing. Fortunately, in the 2021 edition they participated and won again, with an improved tool – AlphaFold2 – capable of predicting the total number of proteins in the human proteome and now, they share the code with everyone.
What was the reaction of the research community to the appearance of all these new structural predictions?
We immediately began to analyze the large amount of data that Google made available to the community and, in fact, the first analyses were published via social networks. It was very fast and everyone was very excited.
Precisely through social networks, a group of 9 very diverse international research groups coordinated to put all this data into a research context and see what impact they could have, how they could be used and what their limitations were.
Now let's recap: why do you need artificial intelligence to do this job? Where is the revolution?
You see, proteins are like a necklace made up of amino acids, placed one after the other. The fact that they are three-dimensionally structured implies that amino acids in the protein that may be far apart in the collar may be very close together in space and this be relevant to the overall function of the protein. This feature – far in the collar, but close in space – is very difficult to assess both by the human brain and by traditional statistical methods, which are linear.
The secret of AlphaFold2 is precisely a very good preliminary work to determine what part of the data is relevant and then apply an artificial intelligence that works in a non-linear way, which is especially good at solving this type of problem.
So, are we facing a new era where more and more work will be done with artificial intelligence? What is the future of these research tools?
Its future is bright! I think it will be just another tool available to researchers. 50 years ago, it was very advanced to have a person working with molecular biology in the laboratory, but today it is the default methodology. In artificial intelligence, I think the same thing will happen: laboratories will have their specialists in artificial intelligence, just as they have bioinformaticians and biochemists.
But artificial intelligence is a bit like a “black box”, often we don't know exactly how it reaches conclusions. How does a scientist, who always wants to understand well the deductive process behind a conclusion, copes with this?
Well, I'm trained in biology and I don't like it at all! However, while it is true that you often do not understand why artificial intelligence has reached the conclusion it offers, there are systems a bit more open, that tell you what factors it has taken into account and allow you to follow its "reasoning".
In any case, on a practical level, if an artificial intelligence tool provides you with useful information that clearly benefits patients, then perhaps you do not need to understand exactly how it was gotten.
I understand… Returning to the structures of proteins: now that we have the complete puzzle, what do we need to know?
Proteins don't work alone! There are hundreds of thousands of proteins working together, interacting and doing their jobs into a cell. Google's predictions don't anticipate which proteins interact with which, for example. Nor can it predict what an altered protein, key to many diseases, would look like. And above all, AlphaFold2 is not able to predict the function of the protein. There are still so many human proteins that we have no idea what they do. There is a lot of work to be done and we are not at the end. Actually, we are still far from it!
Ok, let's talk about your job: how are you using this new structural information in your lab?
With my team we have identified the most important mutations in tens of thousands of cancer patients and, thanks to the structures of AlphaFold2, we can now put them into context. This allows us to see how groups of isolated mutations, which seemed unimportant, become relevant precisely because they affect the structure in space of proteins, something that we could not know before having Google's predictions.
Late-breaking reports indicate that Meta, formerly Facebook, has also gotten in on the game with its ESMFold algorithm. What do you think?
That's right, ESMFold just announced that it has determined a bunch of new structures. It seems that their approximation is faster but less accurate compared to Google’s. We need to wait to see its complete results before telling, but this another computer giant getting in on the field of protein structures reinforces the idea that the future of artificial intelligence is bright and that they will soon be tools commonly used in all laboratories.
Well, it seems that, at the end of the day, Master Asimov was right and that artificial intelligence will end up working side by side with human researchers, to help better understand the most intimate aspects of the diseases we suffer and find a remedy. Hard to see the future is, but just in case, let's get ready for a new batch of Daneel R. Olivaws and Susan Calvins, the imaginary sci-fi characters... or maybe not so much?